(Thanks to Professor Stephen Brown for inspiring the post title.)
Neoliberalism, Collegiality, and Authenticity in a time of Generative AI tools
By Richard Watermeyer, Lawrie Phipps, and Donna Lanclos
We were very pleased to be invited to open the Research and Innovation in Digital Education (RIDE) 2024 conference, at the University of London. The group we spoke to in Senate House and online were welcoming and engaged. This is part 1 of our 2 part blogging about our talk, and the Padlet responses that were collected during the talk and in the Q&A.
While we’ve been working together for more than a year, this was the first time we three had been in a room together. We bring together expertise from Education (Richard), Anthropology (Donna) and Digital Practice and Leadership (by way of Environmental Science and the Royal Navy, Lawrie).
We started our survey project about academic behaviours with generative “AI” tools from a place of concern and curiosity. Collectively we have lived through several cycles of edtech hype; perhaps the earliest one in our memory would be the excitement about putting lectures on CD-ROM. The dropping of generative AI tools (in the form of text generators like ChatGPT and image generators like Mid-journey) into the laps of educators during exam season, December 2022, forced another accelerated hype cycle into our lives (right around the time that people were desperately looking for anything to think about except the ongoing COVID pandemic).
We are not ourselves programmers. We would refer you to computer scientists such as Abeba Birhane and Emily Bender and Margaret Mitchell and Timnit Gebru for a deeper understanding of what LLM tools do (and what is and is not “AI”). Our research focuses on the behaviours of academics who are confronted with these tools in the context of their practices in universities. We were at RIDE to talk about what people’s assumptions about LLMs and generative AI tools can tell us about academic values.
We spoke in turns, with Donna introducing us, then Lawrie setting up the project, and then each of us taking our turn to make our points.
We started with an acknowledgement:
Whilst none of our work was developed using ChatGPT or other Large Language Models, their existence is the subject of our research and we have benefited in carrying out that work.
Therefore we would like to acknowledge that tools like ChatGPT do not respect the individual rights of authors and artists, and ignore concerns over copyright and intellectual property in the training of systems; additionally, we acknowledge that some systems are trained in part through the exploitation of precarious workers in the global south.
You can read the first article to come out of our survey research in Postdigital Science and Education. In a nutshell, we were working with:
- 428 participants
- 284 identifying as academics.
- significant representation from English and pre-1992 universities, predominantly full-time and open-ended contracts.
- Most represented academic positions were Associate Professors (ie, not junior)
- Broad disciplinary representation led by Education (11.9%), Social Studies (10.9%), and Business and Administrative Studies (8.09%).
- roughly even split between academics using and not using GAI tools for work-related purposes.
Regardless of whether people say they are using the tool or not:
- over 70% acknowledged that GAI tools are changing their work methods
- 83% anticipating increased use of GAI tools in the future.
So, people don’t have to be using these tools to experience or perceive the changes in the sector around them.
The focus for our current analysis is the responses to open-ended questions. We had four sets, including:
- For what purposes are you using GAI tools?
- How are GAI tools changing how you work?
- Why are GAI tools not changing how you work?
- Explain why you will be using GAI tools more (or less?)
What is: the landscape of academia in 2024
(Richard)
It seems that GAI tools are becoming seamlessly inserted into the working lives of academics, though often without their knowing. Their normalisation – and the concealment of such by digital industrialists – arrives, however, with the apotheosis of their decline. For many commentators, UK academia is broken; laden with the devastations of a logic of ‘competitive accountability’ through which all academic contributions are rationalised and reduced to prestige points. It is under the rule of competitive accountability that a productivity mania has ensued and a feverish compulsion among academics to commit themselves endlessly – and often injudiciously – as purveyors of output.
As proletariats hitched to an unstoppable production line, academics have become acutely precarious. Their offering is increasingly casualised and replaceable – a condition of a hyper-competitive and contracting labour market. They are simultaneously subject to an epidemic of bullying, harassment and discrimination and degradation by structural inequalities that remain stubbornly persistent. Their working lives are polluted by toxic management which finds no redress and arrested by the anxiety and ambivalence born of lost trust and cultural dissonance. Their health and wellbeing hangs on a gossamer thread and finds little hope of rehabilitation despite the assault of ‘pandemia’ [https://www.tandfonline.com/doi/full/10.1080/01425692.2021.1937058] and flagrancy of universities’ neglect. Instead crisis management has become the de facto norm of institutional leadership. Non-consultative and undemocratic ‘gold command’ ensures that academics’ voice remains marginalised and for the most part impotent. Many are now confronting the intolerability of their working lives by talking with their feet. A diaspora of academics to other international settings or job sectors is in progress. And while UK academia’s allure appears to fade, its future is obfuscated by the rapid accession of GAI.
What Could Be: Algorithmic Conformity
(Lawrie)
Algorithmic conformity is where we see individuals increasingly relying on algorithms in decision-making and content creation leading to uniformity in outputs and outcomes. Generative AI accelerates this process by automating tasks and generating content based on existing patterns and data, making it easier to produce similar outputs at scale and reducing the diversity of ideas and creativity.
When we let algorithms decide what our ideas and outputs look like, it leads us to a world where everything is the same, and human creativity is reduced or lost. Generative AI will push us faster into this situation by doing tasks for us, making everything look and sound alike, and squashing unique ideas.
How would we get to algorithmic conformity?
We already see the proliferation of generative AI tools in academia, influenced by neoliberal pressures for productivity and efficiency. As academia’s shift toward more metrics prioritises quantity of output over quality, the use of these tools for content generation becomes a compelling solution for under pressure academics.Eventually we see the erosion of academic values and practices because of emphasis on productivity and competition. And eventually techno-solutionism wins out with GAI seenI as a means to cope with the intensification of academic work and the precarity it engenders, rather than addressing underlying systemic issues.
Imagine 10 years from now if this pathway were to continue and accelerate. We lose rigour, the quality and authenticity of academic work, as GAI content becomes more prevalent, disappears. Academic work becomes more intensified, while the GAI tools initially relieve some burdens, senior managers see this “freed up time as a capacity to be filled”, leading to even higher expectations for output. Scholars start to use the tool as a substitute for real conversations,they stop working collaboratively, and even consider the tools as co-authors. The differential access to, and the privileged use of GAI tools, where the early adopting global north, and senior established academics have monopolised their use, have exacerbated existing disparities across academia
Inevitably we see a loss of Academic Autonomy. An over dependence on GAI for research and content creation has diminished human intellectual contribution, leading to a homogenisation of thought and research. Academics become devalued, GAI is thought to be capable of performing increasingly complex academic tasks, and the perceived value of human scholars diminish. Instead of conversing with colleagues, bots are used for Socratic dialogue, and prompt engineers write research questions. Eventually in pedagogic research, we see people discussing “machine learning styles” of students.
“But what about the good stuff?” (we hear that a lot from the AI apologists)
We must remember, that at this point, the wide scale availability of Generative AI is less than 2 years old, and yet in Academia alone we have “AI Gurus” and “experts” telling us all about the tools. None of these gurus have done the research of exposing these tools to a cohort of students through their whole university experience, and into employment and looked at the impact of the tools on their learning. NONE. These tools have been deployed by people looking to exploit and profit from the market.
Generative AI is not EdTech. It was not designed with your teaching in mind, nor student learning. AND how do we have experts when it is less than 2 years old
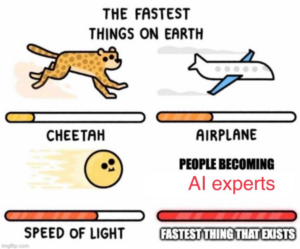
What Should Be: Humanity
(Donna)
We’d like to segue now from the dystopia that Lawrie has set out–the logical progression of what will happen if we insist on stripping our humanity from academic processes.
This is my bias as an anthropologist, but it’s also true that academic systems are human systems. The values and practices are human, created by people, shaped by culture and history. The University is an idea and also an artifact, a made thing.
In these late-capitalist times, when extractive logics demand ROI in all things, the narrative of necessary productivity is in tension with human processes of exploration, of knowing, and frequently of not-knowing.
Academic labour takes time and humanity. We need to recognize and value the human mind in academic labour, broadly defined.
Writing is a process
Research is a process
Advising is a process
Thinking is a process
And all of these things happen in the context of relationships with other humans. We (ideally) talk about our writing with each other, we plan and conduct research together, we advise and consult people, even when we think alone at some point we share our thoughts (through writing, research, etc).
Some of what we saw in our research was people thinking that they could substitute GAI tools for people in these processes.
“I can generate ideas for writing with …”
“I can work out my train of thought in this article with …”
“I can compose letters of recommendation with…”
We need to be the voices asking these people: WHY WOULD YOU DO THAT
Why, when you could talk to colleagues, why when you need to represent your students with your own words and thoughts and feelings WHY when academic life is the life of the mind as much as anything else would you outsource the work of your mind to a machine?
If you don’t want to write, then why pretend you are writing by extruding text via these tools? (Rebecca Solnit says, if you don’t want to write, then don’t write!) If you don’t want to paint then why pretend to generate a painting? If you do want to do those things, the difficult part where you are 1) bad at it and 2) need to learn how to be better are non-negotiable. That’s true no matter where you are in the academic hierarchy, or life generally.
Consider asking why these tools are useful? Consider saying no? Consider what you are trying to do, and whether it should be done with people? If you can’t do it with people, is this really a solution? Where are the people you should be working with?
Is the problem that people are isolated? The solution is not machine learning. It’s battling isolation.
If you are outsourcing it, you likely don’t value it. Like institutions that hire adjuncts for teaching labour instead of full time faculty.
Think as well about the power dynamics currently in universities that make the people saying they want to use the tools more or less likely to be approved of. When white men get called “innovators” for using these tools, are the rest of us perceived in the same way? Or are we “cheating” the way so many say students are?
We want to point to the work of Audra Simpson on ethnographic refusal, as well as Simone Browne’s work on surveillance, sousveillance, and refusal.. A refusal is not just saying no, and leaving the system intact. A refusal is rejecting the framework altogether. It’s not just saying “I’m not going to use LLMs for my academic work” but rather: “I do not recognize the logics that require me to use LLMs for my academic work.”
Do not mistake the tool for the humans who made the tool. Do not make the error that many museums made in conflating the artifact for the humans who created them. The Victorian museum cases full of collected items we see at the Pitt-Rivers, for example, are not the same things as a culture or a people.
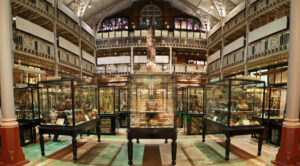
We are worth more than our productivity. If these tools seem like things to use instead of our own minds, why even are we here? What are we doing?
These tools are not currently, nor will they be used for the common good, but rather for the benefit of institutions (and corporations) that are uninvested in human labour and the affordances of human connections.
We are arguing for the value of: human mess. Human tentativeness. Human unfinished business. We are not the sum of the products we generate. We don’t need to do the work for those who want to reduce us in that way.
References and Further Reading
Bender, Emily M., Timnit Gebru, Angelina McMillan-Major, and Shmargaret Shmitchell. “On the dangers of stochastic parrots: Can language models be too big?🦜.” In Proceedings of the 2021 ACM conference on fairness, accountability, and transparency, pp. 610-623. 2021.
Birhane, Abeba. “Automating ambiguity: Challenges and pitfalls of artificial intelligence.” arXiv preprint arXiv:2206.04179 (2022).
Birhane, Abeba. “Algorithmic injustice: a relational ethics approach.” Patterns 2, no. 2 (2021).
Browne, Simone. (2015). Dark matters: On the surveillance of blackness. Duke University Press.
Bryant, Peter. (2022) ‘…and the way that it ends is that the way it began’: Why we need to learn forward, not snap back (blogpost) Peter Bryant: Postdigital Learning. November 4.
Garcia, Antero, Charles Logan and T.Philip Nichols, (2024) “Inspiration from the Luddites: On Brian Merchant’s ‘Blood in the Machine.’” Los Angeles Review of Books, January 28.
Järvinen, M., & Mik-Meyer, N. (2024). Giving and receiving: Gendered service work in academia. Current Sociology, 0(0). https://doi.org/10.1177/00113921231224754
https://journals.sagepub.com/doi/abs/10.1177/00113921231224754?journalCode=csia
Medrado, A., & Verdegem, P. (2024). Participatory action research in critical data studies: Interrogating AI from a South–North approach. Big Data & Society, 11(1). https://doi.org/10.1177/20539517241235869
Simpson, A. (2007). On ethnographic refusal: Indigeneity,‘voice’ and colonial citizenship. Junctures: the journal for thematic dialogue, (9).
Watermeyer, R,. Phipps, L., & Lanclos, D. (2024) ‘Does generative AI help academics to do more or less?,’ Nature https://doi.org/10.1038/d41586-024-00115-7
Watermeyer, R., Phipps, L., Lanclos, D. Knight C (2023) Generative AI and the Automating of Academia. Postdigit Sci Educ https://doi.org/10.1007/s42438-023-00440-6
Williams, Damien Patrick. “Any sufficiently transparent magic…” American Religion 5, no. 1 (2023): 104-110.
Williams, Damien Patrick. “Bias Optimizers.” American Scientist 111, no. 4 (2023): 204-207.
[…] have already posted our opening keynote for RIDE […]