By Donna Lanclos, Lawrie Phipps, and Richard Watermeyer
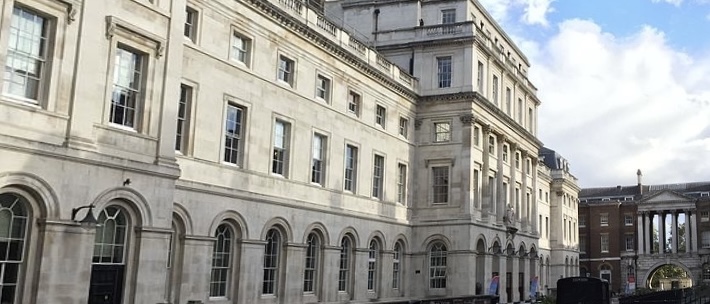
We were pleased to be invited by Darren Moon, conference co-chair and Senior Learning Technologist, Digital Education Team, LSE Eden Centre for Education Enhancement, to address the folks at the Academic Practice and Technology 2024 conference, at King’s College’s Strand Campus, on June 25th.
This is the second talk we’ve given about work that started about two years ago. Richard, having been running a project looking at the digital transformation of higher education in an international context, approached Lawrie and said, “speaking to loads of people heading up digital education and units around the world specifically in elite institutions in the UK Australia US or UN–the thing that keeps coming up (unsurprisingly, Chat GPT just being published at that stage) was the the impact of this on academic communities.” Richard’s question to Lawrie was, what do we know about the way in which academics are utilizing this technology and the impact of on their practices of that use? We turned around and said, we don’t know anything about it, which was the confirmation that we got from many other people working across the globe in this area that kick started our project, the three of us (including Donna) leading on an investigation into academic practices involving generative AI tools, building on previous work from each of us on academic digital practices, and transformation in higher education.
In 2019, Donna had the chance to address the APT room, and spoke at length on the need to recognize and respond constructively to technology refusal among academic staff and students. This talk, in 2024, takes refusal as a starting point, and moves towards an interrogation of what consent means, in this time of embedded GAI tools in institutionally provided systems.
We (Richard and Lawrie, Donna was not in the room this time around) began with our now usual acknowledgement:
Whilst none of our work was developed using ChatGPT or other Large Language Model, their existence is the subject of our research and we have benefited in carrying out that work.
Therefore we would like to acknowledge that the makers of LLM tools like ChatGPT and LIMs like Midjourney do not respect the individual rights of authors and artists, and ignore concerns over copyright and intellectual property in the training of systems; additionally, we acknowledge that some systems are trained in part through the exploitation of precarious workers, especially in the global south.
In November of 2022, Open AI published ChatGPT.
On the 1st of December, 2022, Ars Technica published a piece calling ChatGPT “amusing.” A week later, Ian Bogost was in the Atlantic declaring ChatGPT “Dumber than you Think.” And a few days after that, Fortune published an article declaring it “the most important news event in 2022.”
In 2024 It doesn’t matter how skeptical you are, or used to be, about these tools, they are becoming ubiquitous, embedded into every aspect of what we do online and with digital tools.
Not even two years later we are surrounded by products and platforms in which manufacturers have embedded so-called “AI” tools.: OpenAI’s GPT4o; Meta’s Code Llama79; Microsoft’s Copilot77; and Google DeepMind’s Gemini.
In addition to MS suite, Adobe, and Google products, Gen AI is being embedded into health care software, cars, and even Apple is doing it across its products, with its own proprietary GAI tools.
We thought early in 2023 that it was important to see how academics were using and thinking about the tools, given the hype cycle.
Our research in 2023 on academic uses of GAI tools tried to provide an opportunity for people to tell us why they do or do not (or would or would not, as it was relatively early in the hype cycle) use these tools. The respondents to that survey were largely senior academics, we realized quite early on that we did not hear much at all from early career people (more on that later).
We had 428 participants. 284 of them identified as academics, significant representation were from English and pre 92 universities actually, which was interesting. Most of them were from the older universities that got in touch. The most represented positions were associate professors, not the junior staff. Not people that have just started out in their career, most of them have been in their career for a while. And we had quite a good disciplinary representation but a fairly even split across academics who are using and not using it.
47% of them said that they weren’t using GAI tools at the time of our survey. So about half of the people that we surveyed said, no, I’m not using them, but over 70% of them acknowledged that the tools were having an impact on the work that they did. So even though they weren’t using them, they were seeing things around them that it was going to have an impact on them as well as those that may not ever want to use them, 83% said, yeah, I can see how in the future these tools are gonna impact on what I do on my practice, whether or not I use them. And that’s really interesting–that even if you want to opt out at that point, they still feel the impact.
We wrote in our first article about the ways that academics who responded to our survey say they are and would use GAI tools in their work, and why (and also, why that’s a problem). If you are interested you can read about that open access at Postdigital Science and Education.
For this talk, we wanted to start with the nature of academic skepticism, and how and whether they can decide to not engage with these tools.
In 2022 and early 2023 it was still possible to think of not using the tools, at least for the senior academics we heard from in our survey.
The reasons for refusing to use the tools clustered into two main themes: valuing human minds and interactions, and ethical concerns about the companies that build and train GAI tools. One respondent combined these concerns when saying
“I like to use my brain; I believe AI tools provider have ulterior motive (like collecting our data); and really, it’s quicker to just use my brain then having to figure out the right prompt to generate correct content THEN having to vet that content.”
Respondents emphasized the importance of trust in their work, and the ways that GAI tools might interfere with that:
“I have not found a use-case yet that I am comfortable with. Much of my work depends on bonds of trust and understanding between me and the academics I’m supporting. Using GAI seems more likely to create distance. I need to be present to do my job well. I can’t exercise my social and cognitive presences if I’m not the one doing the writing.”
“I don’t believe we should be using it as it takes away the human touch that should be at the core of what we do. “
Others talked about the importance of the academic skills they had worked hard to acquire, and the importance of doing the work yourself, so as to demonstrate that you value not just the work, but the people with whom you do it:
“[I] think that using it to write text is de-skilling yourself. I am paid for my ability to do these things and so I should honour that. I also would feel disrespected/upset if I received an e.g. email/reference letter etc. from someone written by ChatGPT … as if I wasn’t important enough for them to spend time writing it themselves. “
Some pointed out that using such tools doesn’t actually save the time that some say it will:
“Personally I find it takes me more effort to edit/correct a piece of writing than to write it from scratch. I can write quite fast and I find it helps me to organise my thoughts – I would lose that writing structure and thought process if I used AI-tools. “
Ethical concerns included worries about content scraping by GAI companies (and the attendant harm to people whose work was scraped without permission). Respondents also pointed to the profit motives of GAI companies as incompatible with educational values.
“[I] do not want to support unethical companies who have scraped content generated by other people without permission to generate profit for themselves”
“[I have] significant ethical issues with how generative AI is trained, promoted, monetized, etc. (including not wanting to feed my own data into it by using)”
Later in 2023, in light of what we heard from senior academics in our first survey, we decided to target staff who were working in edtech, ed development, and libraries. We had 118 responses, and brought over 53 responses from our previous survey (ie, people who identified as these specific kind of staff). We are still doing a full analysis of the work, but the majority of the responses were from EdTech and EdDev workers, and most of the respondents in those two groups said they were using GAI tools. The library workers who responded seemed the most skeptical of GAI tools, compared to the rest of the respondents.
What did the EdTech and EdDev workers say were the reasons they needed to use GAI tools?
“[I am] exploring ways in which we can recommend use of AI tools to academics
Some academic staff want to know about these tools, so supporting them in this has been added to my work.”
“Some academic staff want to know about these tools, so supporting them in this has been added to my work.”
“To try out assessment questions and generate a variety of content, so that I can then advise academic colleagues on its use.”
“to demonstrate their functionality among colleagues. As an academic staff developer with expertise on technology enhanced learning, I am delivering some sessions to academic staff promoting the thoughtful and responsible use of these tools aiming to raise their AI literacy levels”
The responses boil down to a lot of : “people are using it, I need to help them.”
What is missing is, “these tools are useful, I need to help people learn how to use them.”
We would ask: are these tools useful?
We need to think critically about what saying yes to a tool might mean. Can we assume that if people are using the tools, it’s because they are actually useful and good? Especially given that these are being embedded into tools that have a long history of being integrated into people’s workflows–like MS tools, the Google Suite, Adobe–what power do people have to simply not use these tools?
We need to talk about the content in which these tools are being framed as “useful” to academic workers. People are using the tools because they are being told they are efficient and good and necessary
In our first survey, one of our respondents laid out the context, the state of higher education in the UK, in these stark terms:
“UK HE is utterly broken with an intrinsically corrupt poorly led business model driven by pseudo-metrics, worsening mediocrity of both academics and students as a consequence, all caused in great part by systematic attacks from multiple governments of late, and with Brexit obviously making it all much worse.”
What we’ve documented and described and theorized in our 2024 article is this valorisation and and normalization of productivity mania. We are constantly chasing for the next hour.
We have staff precarisation and casualisation, and a hyper-competitive labour market, with far more applications for jobs than there are openings. There is additionally extreme workforce inequality, with racialized and gender minoritized people facing limited pathways, many ceilings, and unequal labor burdens.
Once people are in academia, there is an epidemic (perhaps we should say it is endemic) of bullying, harassment, and racial, class, and gender discrimination. People are working in contexts with low trust, and toxic management.
We still see not only amongst our students but our staff, a mental health crisis and that mental health crisis exacerbated by the experience of the pandemic and institutional responses to the pandemic in many ways characterized by a situation of persistent crisis management. And what we’ve elsewhere described as a context of or condition of pandemia, everything from the continuance of long COVID , and the continual breakdown of trust.
We have a work reorganization, in that many of us are now finding ourselves permanently working remotely at a distance from our university campuses in ways that we might not have done before, which has significant implications for our work based communities.
We also have a hostile policy environment and a funding crisis which is engulfing the sector and for which there seems to be little to no policy response or appetite to even get it on the political agenda.
We’re also facing the threat of sector contraction and with increased stratification across our institutions and major workforce attrition, we’re seeing a significant departure of UK academics to other international settings, partially caused by Brexit but also by the worsening conditions within our campuses and an overall sense that the allure of working within UK academia is fading.
And then we’ve got all of this wrapped up in an investment in prestige –higher education is a prestige economy and a sense of value dominated by competitive accountability, the pursuit of the constant accumulation of different forms of positional goods: funding, status within ranking tables, and so on.
And all of this is at the point where we were ensnared in the headlights of generative artificial intelligence.
Let’s remember again the timeline we are dealing with. The trauma of the global pandemic that started in 2020 had not yet finished with us in November of 2022. (it’s still not finished with us).
And in the middle of the trauma, of the death and fatigue and weariness of (in education) dealing with the logistics of tech that could connect us (which contributes to the fatigue as much as it might help sometimes), the venture capitalists of OpenAI thought it would be fun to drop ChatGPT onto us, during exam season, in the autumn of 2022.
For some it was another thing to exhaust and harm us. For others it was a marvelous distraction from the things that exhaust and harm us.
The problem is that this tool can also be a thing that exhausts and harms us, because of the systems in which we work.
We are reminded of the example of students who don’t want to be tracked via the VLE, or other campus systems (parking surveillance, swiping into buildings or classrooms, etc). What happens when they ask not to be tracked? They are told they can either do the work as it’s assigned, in the VLE, or not do the work. They can assimilate, or obstruct (and not get a degree). Where is the third choice? Where is their ability to refuse to be surveilled, as a further price for their education?
Are students who don’t say no to this omnipresent surveillance, saying yes? Why would we assume that, given how hard it is to say no?
And, to bring it back to GAI tools–why would we assume that use of these tools is the same thing as consenting to the full implications of saying yes?
Are academics saying yes because they have to?
Or are they saying yes because they are afraid of what happens if they don’t?
Are people saying yes with enthusiasm because of the tool or because of what they need help with, and the tool seems to be the only choice??
The narrative of increased productivity and efficiency suggests that the decision to use GAI tools in academic workflows is “obvious”– that its voluntary application becomes a non-choice. It is such non-choice that will, we speculate, drive its proliferation and also the potential of its albeit indirect co-option as a means of labour exploitation.
We consider the prospective ubiquity of GAI tools in academia, from the perspective of its silent co-option as a technology of control, to be forcing the capitulation of academic autonomy to managerial intimidation.
We started off talking about the responses of senior academics to the early days of wide availability of GAI tools. A substantial percentage perceived themselves not only as willing but able to say No to these tools. They also clearly felt themselves able to refuse some of the framings of the tools as “essential” to their work. This is because they are: senior. They have achieved success in their fields, they are comfortable and have acquired the habits and signifiers of academic practice before the presence of GAI tools, and therefore are not beholden to them.
EdTech and EdDev workers have far less power in the system, and certainly less prestige than academic staff. It’s telling that their “Yes” to the tools is about helping people learn how to use them. The question of Why we might encourage people to use them seems to be answered most loudly by the managerial priorities of the quantified academy. People are being encouraged to produce more, but not necessarily better.
We are going to continue to dig into our recent survey data to learn more about what these workers have to say.
And what about Early Career Researchers? What about students? Frankly, we’re worried about them. They are just starting to acquire their academic habits. They are still developing their voices and their networks. What will be cut off, cut short, never manifested, what structural harms will be amplified, with the imposition of GAI onto their practices, and ECRs with little to no power to refuse? We are starting a new research project specifically on the practices and priorities of ECRs in the UK, with some of these questions in mind.
The hype cycle provides many alleged benefits of GAI tools to cite. We have discussed the ways that those alleged benefits might not accrue equally.
We should further contrast those alleged benefits with the harms we know are already happening; not just amplification of neoliberal harms to education, but also water crisis, the human harms of dataset cleaning by workers in Kenya and elsewhere, and sustainable energy backsliding, for example keeping fossil fuel power online longer than planned to meet the needs of GAI.
Are we informed? Are we consenting?
Says who?
Is it worth it?
FURTHER READING
Emily M. Bender, Timnit Gebru, Angelina McMillan-Major, and Shmargaret Shmitchell. 2021. On the Dangers of Stochastic Parrots: Can Language Models Be Too Big? 🦜. In Proceedings of the 2021 ACM Conference on Fairness, Accountability, and Transparency (FAccT ’21). Association for Computing Machinery, New York, NY, USA, 610–623. https://doi.org/10.1145/3442188.3445922
Birhane, Abeba. “Automating ambiguity: Challenges and pitfalls of artificial intelligence.” arXiv preprint arXiv:2206.04179 (2022).
Birhane, Abeba. “Algorithmic injustice: a relational ethics approach.” Patterns 2, no. 2 (2021).
Garcia, Antero, Charles Logan and T.Philip Nichols, (2024) “Inspiration from the Luddites: On Brian Merchant’s ‘Blood in the Machine.’” Los Angeles Review of Books, January 28.
Brew, Mavis; Taylor, Stephen; Lam, Rachel; Havemann, Leo and Nerantzi, Chrissi (2023). Towards Developing AI Literacy: Three Student Provocations on AI in Higher Education. Asian Journal of Distance Education, 18(2) pp. 1–11.
Bryant, Peter. (2024) “The Mirror University I– The Cadence of Crisis.” blogpost, https://www.peterbryant.org/the-mirror-university-i-the-cadence-of-crisis/
Chow, Andrew, (2024) “How AI is fueling a boom in Data Centers and Energy Demand,” TIME, June 12. https://time.com/6987773/ai-data-centers-energy-usage-climate-change/
Gilliard, Chris. “Challenging Tech’s Imagined Future.” Just Tech. Social Science Research Council. March 2, 2023. DOI: doi.org/10.35650/JT.3050.d.2023.
Lanclos, Donna (2023) “The Future Is Bullshit” blogpost, https://www.donnalanclos.com/the-future-is-bullshit-gasta-talk-eden-2023/
Lanclos, Donna (2019), “Listening to Refusal.” blogpost
https://www.donnalanclos.com/listening-to-refusal-opening-keynote-for-aptconf-2019/
Logan, C. (2024). Learning about and against generative AI through mapping generative AI’s ecologies and developing a Luddite praxis. In Lingren, R., Asino, T., Kyza, E. A., Chee-Kit, L., Keifert, D. T., & Suárez, E. (Eds.), Proceedings of the 18th International Conference of the Learning Sciences – ICLS 2024 (pp. 362-369). Buffalo, United States of America.
Mathewson, Tara Garcia (2023) “ He wanted Privacy. His College Gave him None.” The Markup. Nov 30. https://themarkup.org/machine-learning/2023/11/30/he-wanted-privacy-his-college-gave-him-none
Mengesha, L., & Padmanabhan, L. (2019). Introduction to Performing Refusal/Refusing to Perform. Women & Performance: a journal of feminist theory, 1-8.
Perrigo, Billy (2023) “Exclusive: OpenAI Used Kenyan Workers on Less Than $2 Per Hour to Make ChatGPT Less Toxic” Time Magazine, January 18, 2023, https://time.com/6247678/openai-chatgpt-kenya-workers/
Simpson, A. (2007). On ethnographic refusal: indigeneity,‘voice’ and colonial citizenship. Junctures: The Journal for Thematic Dialogue, (9).
Watermeyer, R., Phipps, L., Lanclos, D. et al. Generative AI and the Automating of Academia. Postdigit Sci Educ 6, 446–466 (2024). https://doi.org/10.1007/s42438-023-00440-6
Williams, Damien Patrick. “Any sufficiently transparent magic…” American Religion 5, no. 1 (2023): 104-110.
Williams, Damien Patrick. “Bias Optimizers.” American Scientist 111, no. 4 (2023): 204-207.